Contents
The evolution of AI Agents
5 minutes read
30 March 2025
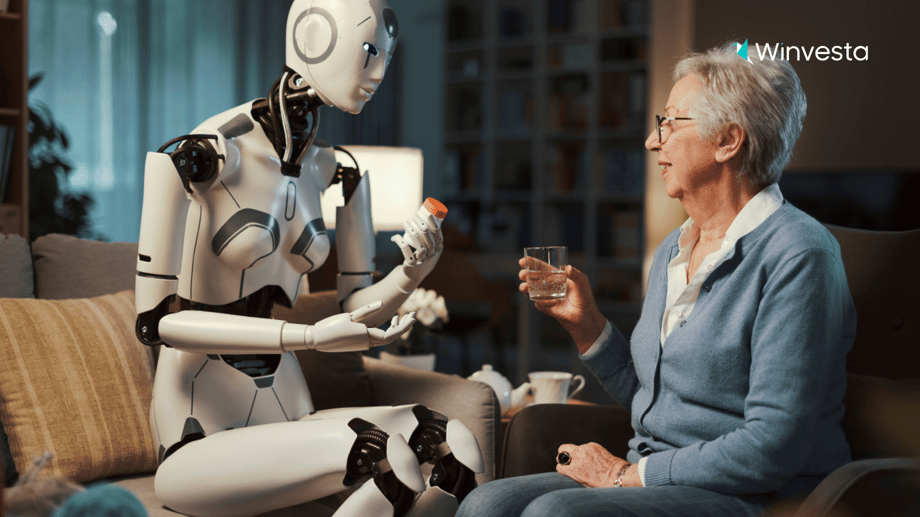
Artificial Intelligence (AI) agents have come a long way. From the humble beginnings of rule-based systems to today's sophisticated, autonomous agents, the evolution of AI is a fascinating journey. These AI systems, once limited to basic tasks, now have the capability to make complex decisions, learn from data, and even collaborate with humans and other AI agents. This transformation has not only revolutionized industries but also changed the way we interact with technology.
In this blog, we’ll explore the history and advancements of AI agents, from the early rule-based systems to the cutting-edge autonomous systems we see today. We'll also take a look at the future of these intelligent agents and the impact they will have on our lives.
A glimpse into the past: Rule-based AI (1950s-1980s)
In the early days of AI, the focus was on creating machines that could simulate human intelligence through simple rule-based systems. These early systems operated on a set of predetermined rules that dictated how they would respond to certain inputs.
Key developments:
- ELIZA (1966): Created by Joseph Weizenbaum, ELIZA was one of the first chatbots. Although primitive, it laid the groundwork for future AI-driven conversational agents by mimicking human interaction through pattern recognition.
- Expert Systems (1970s-80s): AI began to take a more specialized turn with expert systems like MYCIN, which used predefined rules to diagnose medical conditions. These systems were successful in solving problems in narrow domains but lacked the ability to handle complex or dynamic situations.
- PROLOG (1972): A programming language that paved the way for logical reasoning and problem-solving in AI.
Though groundbreaking, these systems had limited flexibility and could only perform tasks they were explicitly programmed for. They lacked true understanding and were unable to adapt to new scenarios.
The rise of machine learning (1980s-2000s)
As we entered the 1980s and 1990s, AI shifted from static rule-based systems to more dynamic models that could learn from data. The advent of machine learning (ML) made AI agents more intelligent and adaptable.
Key advancements:
- Reinforcement Learning (1988): Researchers Sutton and Barto introduced temporal difference learning, a key technique in reinforcement learning that helped AI agents improve through trial and error.
- Intelligent Agents (1990s): AI systems started to exhibit autonomy, meaning they could process data, make decisions, and adapt over time.
- IBM Watson (2006): Perhaps the most famous achievement in this era, IBM Watson famously defeated human contestants on the quiz show Jeopardy!, showcasing the potential of AI in processing and analyzing vast amounts of information.
The use of neural networks, especially with the development of backpropagation algorithms, opened the door to more complex, self-improving systems. These systems could learn from their experiences and adapt, making them much more powerful than their predecessors.
Deep learning revolution (2010s)
The 2010s were a pivotal decade for AI, driven by deep learning, an advanced subset of machine learning. With increased computational power and access to massive datasets, AI agents became more capable than ever before.
Key milestones:
- AlexNet (2012): This breakthrough in image recognition demonstrated the power of deep neural networks, ushering in a new era of AI applications in computer vision.
- Virtual Assistants: The launch of Siri (2011), Alexa (2014), and Google Assistant signaled a new era in AI interaction. These AI assistants use natural language processing (NLP) to understand and respond to human queries, setting the stage for more intuitive and conversational AI.
- Self-Driving Vehicles & Robotics: AI agents moved from software into the physical world. Companies like Tesla, Waymo, and others began using AI to make real-time, high-stakes decisions in self-driving vehicles.
This decade also saw AI agents being deployed in industries ranging from healthcare to finance, significantly increasing their impact. The ability to process vast amounts of unstructured data—such as images, speech, and text—enabled these agents to handle far more complex tasks.
The era of large language models (Late 2010s-Early 2020s)
By the late 2010s, AI agents took another giant leap forward with the introduction of large language models (LLMs) like GPT-3. These models excel in understanding and generating human-like text, leading to a surge in conversational AI.
Key developments:
- GPT-3 (2020): This large language model, developed by OpenAI, became famous for its ability to generate coherent and contextually accurate text. It demonstrated the potential for AI to engage in more complex conversations and produce content that was indistinguishable from human writing.
- ChatGPT (2022): The introduction of ChatGPT brought conversational AI to the masses, allowing users to interact with AI in a natural, intuitive manner.
- GPT-4 (2023): The release of GPT-4 pushed the boundaries even further, with improvements in text generation, logic, and reasoning.
Large language models are able to process vast amounts of text data, allowing them to generate human-like responses across a wide range of topics. This has revolutionized everything from customer service to content creation, making AI more accessible and practical for everyday tasks.
The rise of agentic AI (Present and Future)
Today, we find ourselves entering a new era: the era of agentic AI. These AI systems not only process data and provide responses but can also make independent decisions, engage in long-term planning, and collaborate with other AI agents to tackle complex problems.
Key characteristics of agentic AI:
- Enhanced Reasoning Abilities: Modern AI agents can analyze complex situations and make nuanced decisions, whether in healthcare, business, or governance.
- Multi-Step Task Handling: Today’s AI agents can break down large, complicated tasks into smaller steps and execute them autonomously, making them incredibly efficient.
- Multi-agent Collaboration: AI agents are learning to collaborate with one another, mimicking human teamwork to solve problems that would be difficult for a single agent to tackle alone.
- Integration with IoT: AI agents now interact with smart devices and sensors, extending their reach beyond digital environments into the physical world.
These agents are capable of more than just performing tasks—they can make decisions in real-time, adapt to new information, and handle previously unimaginable complexities.
The impact and applications of autonomous AI Agents
The rise of autonomous AI agents has had a profound effect on a variety of industries:
- Business Operations: AI agents are streamlining project management, optimizing workflows, and automating routine tasks like data entry.
- Customer Service: AI chatbots with advanced NLP are providing more accurate and personalized customer support.
- Healthcare: From health tracking to real-time mental health support, AI agents are playing a significant role in healthcare innovation.
- Finance: AI systems are transforming the finance industry by managing portfolios, providing investment advice, and automating trading decisions.
- Governance: AI agents assist policymakers by providing real-time insights and recommendations on complex issues such as climate change, regulations, and economic strategy.
Challenges and future directions
While the future of autonomous AI agents is promising, several challenges remain:
- Ethics: How can we ensure that AI agents are used responsibly, especially in high-stakes applications like healthcare and governance?
- Transparency: There is an increasing need for AI systems to be explainable, allowing humans to understand their decision-making processes.
- Security and Privacy: With AI agents gaining more autonomy and access to sensitive data, ensuring robust security measures is essential.
As AI agents evolve, we can expect to see even greater integration into our lives. Their ability to understand human needs, adapt to changes, and work alongside humans will shape the future of work and technology.
Meet your new AI teammate
The future of work is here. AI that works with you, not instead of you.
- Never stop scaling
- 24/7 autonomous execution
- Scale your productivity with AI.
The evolution of AI agents from basic bots to autonomous systems has been nothing short of revolutionary. As these systems continue to improve, they promise to change the way we interact with technology, making our lives more efficient, productive, and connected. However, it’s essential that we address the ethical, privacy, and security challenges to ensure AI’s positive impact on society. With continued innovation, AI agents will shape the future, transforming industries and enhancing human-AI collaboration.