Contents
What is Agentic AI?
6 minutes read
30 March 2025
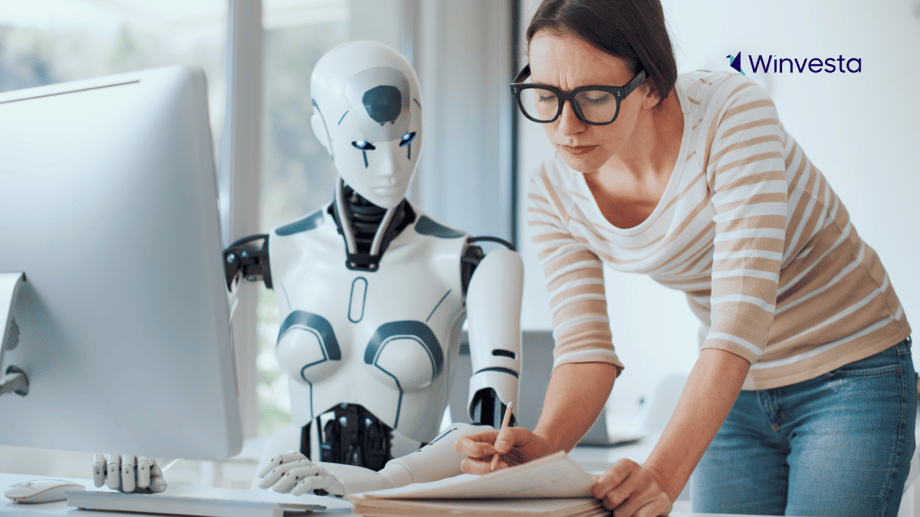
Did you know that agentic AI, also known as agent AI, is projected to automate up to 30% of work hours by 2030? Unlike traditional AI systems, agentic AI uses sophisticated reasoning and iterative planning to solve complex problems without constant human guidance. This autonomous capability, powered by intelligent agents in artificial intelligence, is already showing impressive results across industries - marketers save an average of three hours per content piece, while service professionals report significant improvements in customer interactions.
You might wonder what makes agentic AI so powerful. It's the ability to make decisions, work independently, and tackle multi-step challenges through AI agents that can plan and evaluate their progress. These autonomous AI agents can handle both routine operations and complex analytical tasks, allowing your team to focus on strategic initiatives. In this article, you'll discover how agentic AI works, its various types, real-world applications, and how it compares to other AI systems.
Understanding the fundamentals of agentic AI
Agentic AI represents a significant advancement in artificial intelligence, combining autonomous decision-making with adaptive learning capabilities. The first thing to remember is that these systems operate through a probabilistic approach, analyzing patterns and likelihoods to make decisions rather than following fixed rules.
Core concepts and principles
The foundation of agentic AI rests on three fundamental principles. Additionally, these systems exhibit autonomy in initiating and completing tasks without continual oversight. Furthermore, they employ sophisticated reasoning for context-based decisions and utilize reinforced learning to evolve through environmental interactions. The system's ability to comprehend complex instructions enables efficient execution of multi-step processes.
How agentic AI differs from traditional AI systems
Traditional AI systems follow predefined rules and operate within structured environments. Consequently, agentic AI breaks through these limitations by:
- Processing real-time data through sensors and input devices
- Applying advanced decision-making frameworks
- Executing tasks autonomously based on processed information
- Adapting behaviour through continuous learning.
Key components of the agentic architecture
The architecture of agents in AI comprises interconnected modules that enable intelligent behaviour. Moreover, the perception module processes sensory input and extracts meaningful features. The cognitive module handles goal representation and planning, generating strategies based on the agent's capabilities. The action module controls physical or virtual responses through actuators, implementing selected actions to achieve desired outcomes.
The learning module forms the system's core, incorporating reinforcement learning for experience-based improvements and unsupervised learning for pattern discovery. These components work together seamlessly, enabling the system to perceive, process, decide, and act effectively in complex environments.
Types of AI agents and their roles
AI agents form the backbone of agentic AI systems, each designed with specific capabilities and roles. Specifically, these agents range from simple task executors to complex decision-makers, adapting to various scenarios and requirements. Understanding the different types of agents in AI is crucial for effective implementation.
Task-specific agents vs general-purpose agents
Task-specific agents excel at focused operations, offering enhanced efficiency and lower costs. These agents process particular functions with precision, such as data analysis or workflow automation. Essentially, they deliver faster performance and reduced latency compared to their general-purpose counterparts.
General-purpose agents handle diverse tasks but require more resources and complex programming. Primarily, they shine in scenarios demanding flexibility and broad capability ranges, though at higher computational costs.
Learning and adaptive agents
Learning agents stand out through their ability to improve performance over time. These intelligent agents in artificial intelligence:
- Analyze patterns and outcomes
- Adjust strategies based on feedback
- Store solutions for future reference
- Self-correct through iterative refinement
Adaptive agents enhance their capabilities through reinforcement learning and neural architecture modifications. They continuously refine their responses by integrating new information and adjusting to environmental changes.
Collaborative multi-agent systems
Collaborative Multi-Agent Systems (CMAS) enable multiple autonomous agents to work together toward common goals. Subsequently, these systems divide complex tasks among specialized agents, creating a robust and scalable solution.
The success of CMAS depends on effective communication protocols and coordination mechanisms. Each agent maintains individual goals while contributing to collective objectives, making the system resistant to single-point failures. CMAS tackles tasks that would overwhelm individual agents through this collaborative approach, offering enhanced problem-solving capabilities and improved system reliability.
Comparing agentic AI with generative AI
The fundamental distinction between agentic AI and generative AI lies in their operational approach and core functionalities. Understanding these differences is crucial for determining the most suitable AI solution for specific tasks.
Architectural differences
Agentic AI employs multi-component decision systems that integrate reinforcement learning and decision trees for structured problem-solving. Primarily, these systems process real-time environmental data through sensor inputs, enabling dynamic responses. In contrast, generative AI relies on transformer-based models like large language models (LLMs) and BERT, designed to analyze patterns and create content based on existing datasets.
Capability comparison
The capabilities of these AI systems differ significantly in their operational nature. Agentic AI demonstrates:
- Autonomous decision-making without human intervention
- Real-time environmental adaptation
- Proactive problem-solving abilities
- Goal-oriented task execution
Particularly, generative AI depends heavily on human guidance and prompts to function effectively. Indeed, despite its ability to create impressive outputs using natural language processing, it remains fundamentally reactive, responding only to specific user inputs.
Use case differentiation
Each system excels in distinct applications across industries. Accordingly, generative AI performs exceptionally well in creative tasks, including marketing copy generation, visual content creation, and code development. Nevertheless, agentic AI finds its strength in scenarios demanding autonomous operation, such as self-driving vehicles, robotic manufacturing, and AI-powered logistics.
Similarly, in financial services, generative AI assists with content creation and data analysis, whereas agentic AI handles complex tasks like algorithmic trading and real-time market analysis. The manufacturing sector particularly benefits from agentic AI's ability to adapt to production schedule changes, improving efficiency and reducing downtime.
Real-world applications of agentic AI
Major enterprises across industries implement agentic AI to enhance operational efficiency and customer experience. Primarily, these implementations focus on automating complex tasks and decision-making processes, showcasing the versatility of AI agents in various sectors.
Enterprise implementation examples
BMC recently launched AI agents within their Helix platform, powered by BMC HelixGPT. During implementation, these agents perform diverse roles, from data analysis to knowledge management. The BMC Insight Finder simplifies complex data analysis through natural language interactions, earlier requiring extensive manual effort.
Industry-specific use cases
The healthcare sector presently uses agentic AI for precision medicine and treatment planning. Tempus Labs applies this technology in oncology for personalized cancer care. Financial institutions automate compliance checks and transaction processing, with Goldman Sachs integrating agentic AI into trading platforms.
In cybersecurity, agentic AI addresses the global shortage of professionals, which is four million. These systems detect cyber attacks and help software engineers identify code vulnerabilities. The manufacturing sector benefits through:
- Autonomous equipment monitoring
- Real-time production adjustments
- Supply chain optimization
- Quality control automation
Success stories and case studies
Power Design achieved significant efficiency gains by deploying an AI copilot named HelpBot for employee self-service. Overall, the system handles password resets and device monitoring autonomously, reducing IT workload.
Bud Financial demonstrates another successful implementation in consumer banking. Generally, their AI solution manages money transfers between accounts to prevent overdraft fees and optimize interest earnings. A sizeable Dutch insurer automated approximately 90% of individual automobile claims by integrating AI agents into their claims workflow.
Agentic AI is a transformative force, reshaping how businesses handle complex tasks through autonomous decision-making and adaptive learning. Your organization can benefit from its ability to automate up to 30% of work hours, allowing teams to focus on strategic initiatives rather than routine operations.
Task-specific agents excel at focused operations, while collaborative multi-agent systems tackle more complex challenges through coordinated efforts. Major enterprises have already demonstrated significant gains, from BMC's Helix platform streamlining data analysis to Power Design's HelpBot, which has reduced IT workload.
The technology differs significantly from traditional and generative AI through its proactive problem-solving abilities and real-time environmental adaptation. Success stories across healthcare, finance, and manufacturing sectors prove its practical value. Dutch insurers automated 90% of automobile claims, while Tempus Labs enhanced cancer care through personalized treatment planning.
As AI technology advances, agentic systems will become essential for business growth and operational efficiency. Their ability to learn, adapt, and execute complex tasks autonomously positions them as valuable assets for organizations seeking competitive advantages in an increasingly digital world. By leveraging AI-driven automation and personalized experiences, businesses can create a robust data flywheel effect, continuously improving their operations and customer satisfaction.
Meet your new AI teammate
The future of work is here. AI that works with you, not instead of you.
- Never stop scaling
- 24/7 autonomous execution
- Scale your productivity with AI.
Frequently asked questions about Agentic AI
Disclaimer: The information in this blog about Agentic AI is intended for general informational purposes only and should not be considered as professional, technical, or business advice. While we strive for accuracy, AI technologies are rapidly evolving, and readers should conduct independent research or consult experts before making decisions based on the content provided.
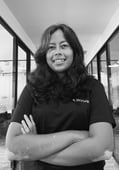
Contributed by Denila Lobo
Denila is a content writer at Winvesta. She crafts clear, concise content on international payments, helping freelancers and businesses easily navigate global financial solutions.